视频Pipeline演示如何基于时间戳或帧号应用标签#
在本示例中,我们将介绍如何使用 readers.video 算子创建一个pipeline,以读取视频及其标签。我们将基于帧号作为输入将标签应用于视频,并读取时间戳和帧号标签以及解码后的视频帧。
有关 readers.video
参数的更多信息,请查阅文档。
首先,让我们导入库。
[1]:
import os
import numpy as np
import tempfile
from nvidia.dali import pipeline_def
import nvidia.dali.fn as fn
import nvidia.dali.types as types
try:
from PIL import Image
has_PIL = True
except ImportError:
has_PIL = False
然后我们定义,在pipeline的每次迭代中,我们想要 4 个批次,并且每个批次的序列长度为 1 帧。我们希望运行 10 次迭代以进行说明。
[2]:
BATCH_SIZE = 4
COUNT = 1
ITER = 10
frame_num_based_labels = True
我们在本示例中使用的视频是一个 10 秒长,25fps,512x512 分辨率的视频,时间戳烧录在视频上半部分的帧本身中,帧号在下半部分。解码帧中烧录的文本可以很容易地用于验证我们确实返回了正确的帧号和时间戳标签。
注意:DALI_EXTRA_PATH
环境变量应指向从 DALI extra repository 下载数据的位置。请确保已检出正确的发布标签。
[3]:
dali_extra_path = os.environ["DALI_EXTRA_PATH"]
file_list_txt = dali_extra_path + "/db/video/test_label.mp4 0 0 100\n"
file_list_txt += dali_extra_path + "/db/video/test_label.mp4 1 100 200\n"
file_list_txt += dali_extra_path + "/db/video/test_label.mp4 2 200 250\n"
tf = tempfile.NamedTemporaryFile()
tf.write(str.encode(file_list_txt))
tf.flush()
定义 Pipeline#
然后我们可以定义一个最小的 Pipeline,它将直接输出 readers.video 的输出。
请注意,我们设置了 file_list_frame_num=True
,这意味着我们上面使用 file_list
提供的标签将被解释为帧号。test_label.mp4 0 0 100
被解释为从帧号 0
到 100
(不包括)应用标签 0
。
但是,如果您希望使用时间戳应用标签,只需设置 file_list_frame_num=False
(默认行为),并提供您希望应用标签的时间戳。例如,如果您希望将标签 1
应用于从 3
秒到 8.5
秒的帧:test_label.mp4 1 3 8.5
在本示例中,我们出于说明目的将 enable_frame_num
和 enable_timestamps
都设置为 True
。您可以根据您的特定用例启用或禁用其中任何一个。
[4]:
@pipeline_def
def video_pipe(file_list):
video, label, start_frame_num, timestamps = fn.readers.video(
device="gpu",
file_list=file_list,
sequence_length=COUNT,
shard_id=0,
num_shards=1,
random_shuffle=True,
initial_fill=10,
image_type=types.RGB,
dtype=types.FLOAT,
file_list_frame_num=frame_num_based_labels,
enable_frame_num=True,
enable_timestamps=True,
file_list_include_preceding_frame=True,
)
return video, label, start_frame_num, timestamps
可视化结果#
我们将使用 matplotlib 来显示我们获得的帧和相关的标签。
[5]:
%matplotlib inline
from matplotlib import pyplot as plt
import matplotlib.gridspec as gridspec
[6]:
pipe = video_pipe(
batch_size=BATCH_SIZE, num_threads=2, device_id=0, file_list=tf.name
)
pipe.build()
gs = gridspec.GridSpec(ITER, 1)
for i in range(ITER):
sequences_out, label, start_frame_num, timestamps = pipe.run()
sequences_out = sequences_out.as_cpu().as_array()
label = label.as_cpu().as_array()
start_frame_num = start_frame_num.as_cpu().as_array()
timestamps = timestamps.as_cpu().as_array()
batch_sequences = sequences_out[0]
sample_frame = batch_sequences[0]
if has_PIL:
im = Image.fromarray(sample_frame.astype("uint8"))
fig = plt.figure(figsize=(16, 64), facecolor="#76b900")
plt.subplot(gs[i])
plt.axis("off")
plt.title(
"label=" + str(label[0][0]) + "\n"
"frame number=" + str(start_frame_num[0][0]) + "\n"
"timestamp=" + str(round(timestamps[0][0], 2)),
fontsize=20,
)
plt.imshow(im)
plt.close()
tf.close()
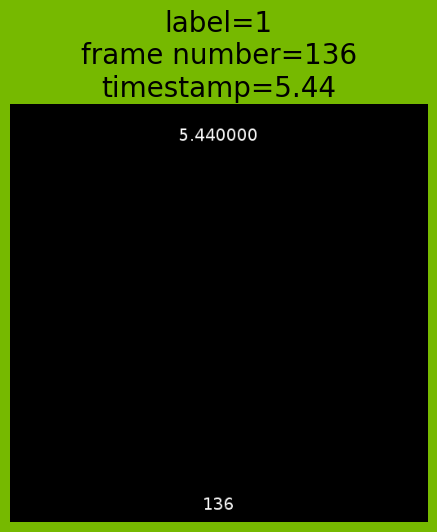
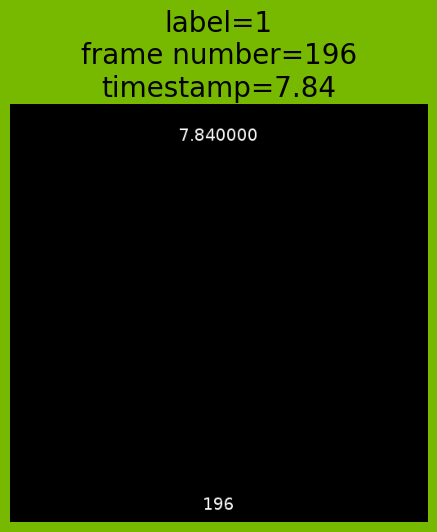
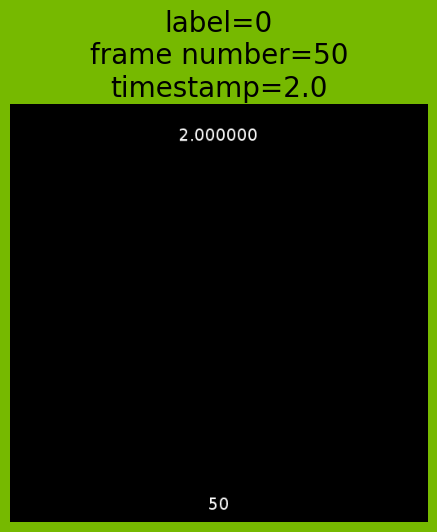
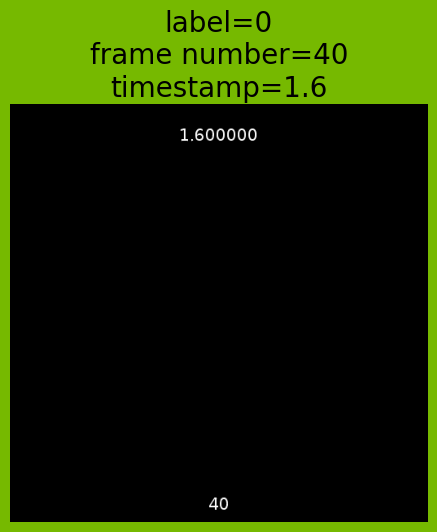
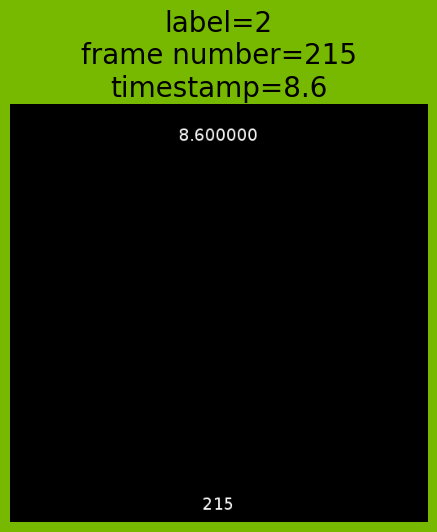
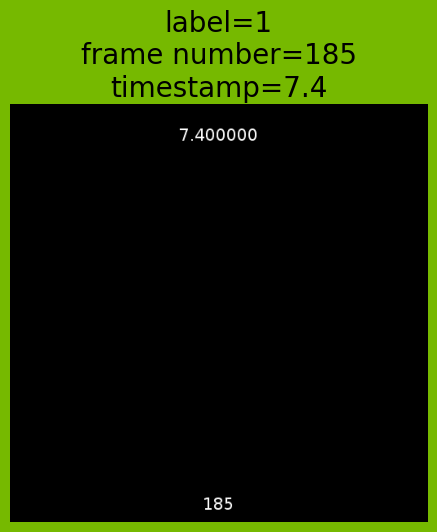
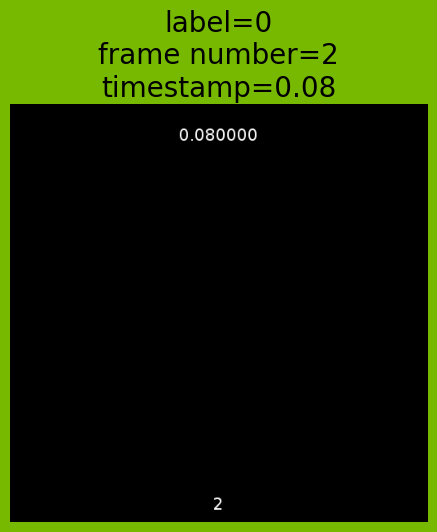
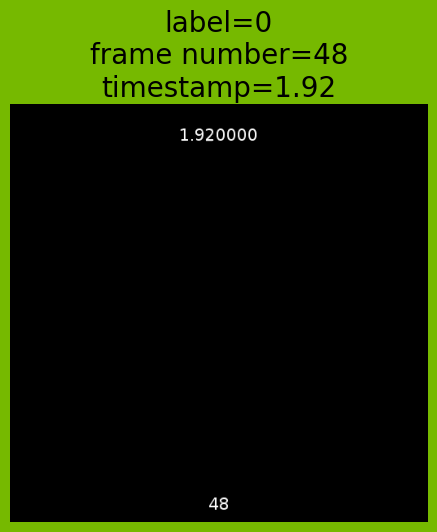
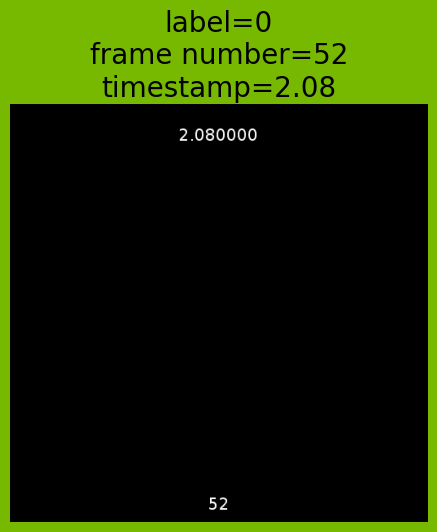