DALI 中的条件执行#
DALI pipeline 表示数据处理图 - 数据由一个或多个 DALI 操作符处理,这些操作符是无条件运行的。通常,在训练期间,这些操作由随机值参数化 - 例如,图像可以按随机角度旋转。要跳过此类操作,用户必须确保提供中性参数 - 在旋转的情况下,它需要是 0 度旋转角。
找到这样的配置可能不切实际,并且提供中性值的操作符可能仍然会调用一些计算。
DALI 允许在 pipeline 定义中使用基于 if
的控制流,使用 @pipeline_def
装饰器。if 和 else 分支将仅针对适用相应条件的样本执行。
让我们定义一个简单的示例,假设我们希望以 25% 的概率将图像旋转一个 [10, 30)
范围内的角度,否则完全跳过旋转。
简单示例#
要启用条件语句,我们需要将 enable_conditionals
标志传递给 @pipeline_def
装饰器。
我们的 pipeline 执行以下操作
读取和解码图像。
准备一个随机谓词
do_rotate
,用作if
条件来决定我们是否要旋转样本。准备用于旋转的随机角度。
有条件地应用(随机)旋转。我们使用 0 值填充以更好地突出显示输出中的旋转。
调整结果大小以对其进行归一化,并返回 pipeline 的输出。
[1]:
from nvidia.dali import pipeline_def
from nvidia.dali.types import DALIDataType
from nvidia.dali import fn
from nvidia.dali import tensors
[2]:
@pipeline_def(
enable_conditionals=True, batch_size=4, num_threads=4, device_id=0, seed=42
)
def rotate_pipe():
jpegs, _ = fn.readers.file(device="cpu", file_root="../data/images")
images = fn.decoders.image(jpegs, device="mixed")
do_rotate = fn.random.coin_flip(probability=0.25, dtype=DALIDataType.BOOL)
angle = fn.random.uniform(range=(10, 30))
if do_rotate:
result = fn.rotate(images, angle=angle, fill_value=0)
else:
result = images
resized = fn.resize(result, resize_x=400, resize_y=400)
return resized
现在,让我们构建并运行 pipeline。
[3]:
pipe = rotate_pipe()
pipe.build()
[4]:
import matplotlib.pyplot as plt
def display(output):
data_idx = 0
fig, axes = plt.subplots(len(output) // 2, 2, figsize=(15, 15))
if len(output) == 1:
axes = [axes]
for i, out in enumerate(output):
img = out if isinstance(out, tensors.TensorCPU) else out.as_cpu()
axes[i // 2, i % 2].imshow(img)
axes[i // 2, i % 2].axis("off")
[5]:
(output,) = pipe.run()
display(output)
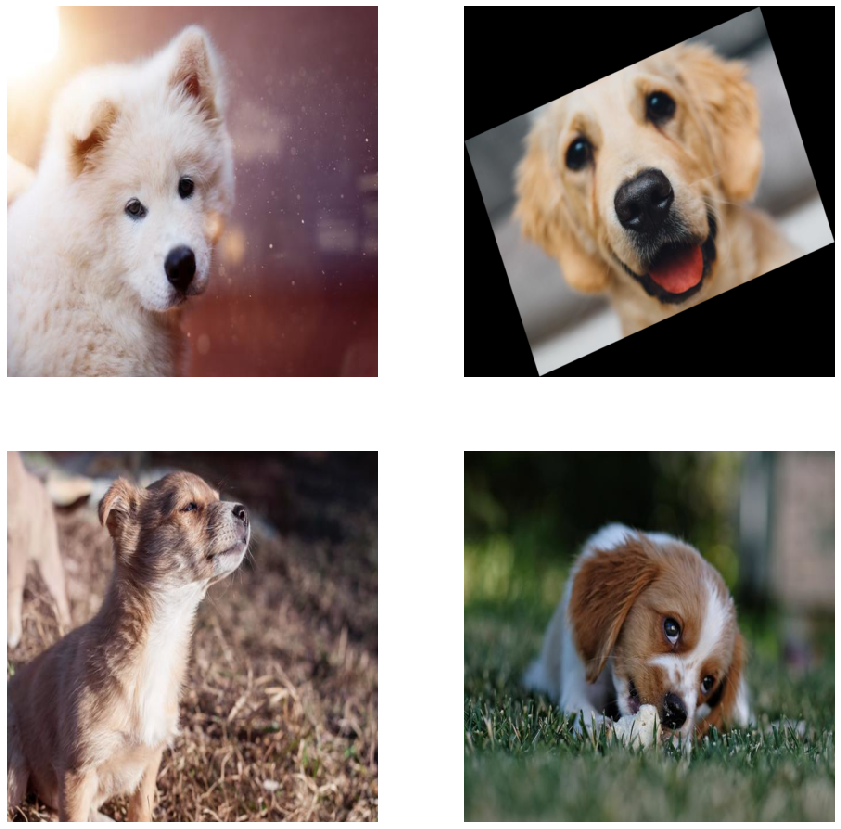
正如我们所见,在生成的 4 个图像批次中,其中一个图像按随机角度旋转。
语义#
虽然 DALI 在批次上运行,但我们编写的代码可以理解为它一次在一个样本上运行。
要求#
在使用 DALI pipeline 中的条件语句时,需要满足以下几个要求
if
条件必须是 DALI DataNode,并且底层数据的数据类型必须为BOOL
或其他数值类型,这些类型可以根据 Python 语义评估为 True 或 False。
这样做的原因是条件必须在 DALI pipeline 运行期间可跟踪,并且必须表示一个批次 - 每个样本都获得其关联的条件值。
如果条件是其他 Python 值,则代码将使用常规 Python 语义执行,仅采用一个分支,并且 DALI pipeline 图不会捕获任何条件操作。
if
条件必须是 CPU 数据批次。条件语句的“输出”变量必须在每个分支中定义。输出变量是在条件分支范围内定义或修改并在条件语句之后使用的那些变量 - 在示例中,它是
result
变量。
作为替代方案,我们可以将条件语句重写为
result = images
if do_rotate:
result = fn.rotate(images, angle=angle, fill_value=0)
每个分支的输出必须兼容 - 在条件语句之后,来自 DALI 图中所有代码路径的相应输出将合并回一个批次,因此每个分支的数据类型、维度和布局必须匹配。
这种
if
语句的所有输出变量都是 DALI DataNode,可以转换为 DALI 常量,或者这些常量的嵌套结构。例如,NumPy 数组会自动转换为 CPU 常量节点(DALI Constant 操作符 - 它生成一个批次,其中所有样本都是该数组)。嵌套结构是由字典、列表和元组构建的 Python 数据结构 - 在这种情况下,它们可以包含 DALI DataNode 或常量作为其值。结构在分支之间必须匹配 - 例如,我们可以使用相同长度的元组或包含相同键集的字典 - 值可能不同。
一个最简单的例子是字典的单层嵌套
if do_rotate:
output_dict['result'] = fn.rotate(images, angle=angle, fill_value=0)
else:
output_dict['result'] = images
result = output_dict['result']
如果我们在 if/else
分支中使用不同的键名,则无法合并结构,并且会导致错误。
技术细节#
在底层,DALI 利用 TensorFlow 的 AutoGraph 的一个分支来捕获 if
语句,并将它们重写为允许跟踪两个分支的函数。这意味着对于包含 DALI DataNode 作为条件的 if
语句,两个分支都将被执行。我们稍后会看到这一点。
建议使用函数式风格编写 pipeline,因为将条件语句的结果定义为副作用的一种手段(例如将它们分配为类成员并依赖于此类本地状态中存在的值)可能无法正确捕获。
捕获的代码被转换为 DALI 处理图。每个 if/else
对都通过拆分输入批次(在 if
语句外部创建并在其中一个分支中用作操作符输入或在赋值中引用的变量)为较小的批次来实现,在样本子集上运行请求的操作,并将结果合并到输出变量中。
等效 pipeline#
以下是使用内部拆分和合并操作符的功能等效代码,这些操作符实现了条件。请注意,拆分和合并不会引入额外的副本,并允许我们仅在应用条件的部分批次上执行操作符。
[6]:
@pipeline_def(batch_size=4, num_threads=4, device_id=0, seed=42)
def manual_rotation_pipe():
jpegs, _ = fn.readers.file(device="cpu", file_root="../data/images")
images = fn.decoders.image(jpegs, device="mixed")
do_rotate = fn.random.coin_flip(probability=0.25, dtype=DALIDataType.BOOL)
angle = fn.random.uniform(range=(10, 30))
images_true_branch, images_false_branch = fn._conditional.split(
images, predicate=do_rotate
)
angle_true_branch, angle_false_branch = fn._conditional.split(
angle, predicate=do_rotate
)
result_true = fn.rotate(
images_true_branch, angle=angle_true_branch, fill_value=0
)
result_false = images_false_branch
result = fn._conditional.merge(
result_true, result_false, predicate=do_rotate
)
resized = fn.resize(result, resize_x=400, resize_y=400)
return resized
[7]:
manual_pipe = manual_rotation_pipe()
manual_pipe.build()
[8]:
(output,) = manual_pipe.run()
display(output)
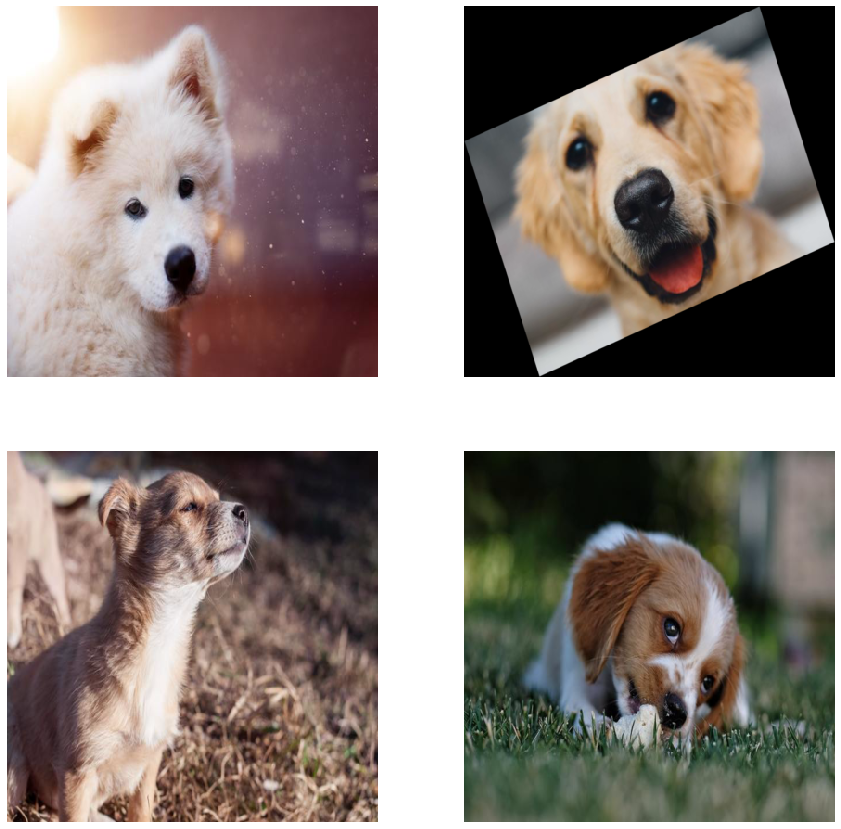
生成器#
当前,使用生成器操作符(如随机生成器和读取器)会导致它们被执行,就像它们在“全局范围”中运行一样,即使它们被放在分支的范围内。将它们用作分支内任何内容的输入将仅采用生成批次的子集,但无论如何都将生成整个批次。
函数#
if
语句的跟踪不限于 pipeline 定义函数。DALI 和 AutoGraph 执行并跟踪用于创建 pipeline 的所有代码,因此可以创建包含条件语句的辅助函数。
[9]:
def random_rotate(images):
angle = fn.random.uniform(range=(10, 30))
return fn.rotate(images, angle=angle, fill_value=0)
@pipeline_def(
enable_conditionals=True, batch_size=4, num_threads=4, device_id=0, seed=42
)
def rotate_with_helper_pipe():
jpegs, _ = fn.readers.file(device="cpu", file_root="../data/images")
images = fn.decoders.image(jpegs, device="mixed")
do_rotate = fn.random.coin_flip(probability=0.25, dtype=DALIDataType.BOOL)
if do_rotate:
result = random_rotate(images)
else:
result = images
resized = fn.resize(result, resize_x=400, resize_y=400)
return resized
[10]:
helper_pipe = rotate_with_helper_pipe()
helper_pipe.build()
[11]:
(output,) = helper_pipe.run()
display(output)
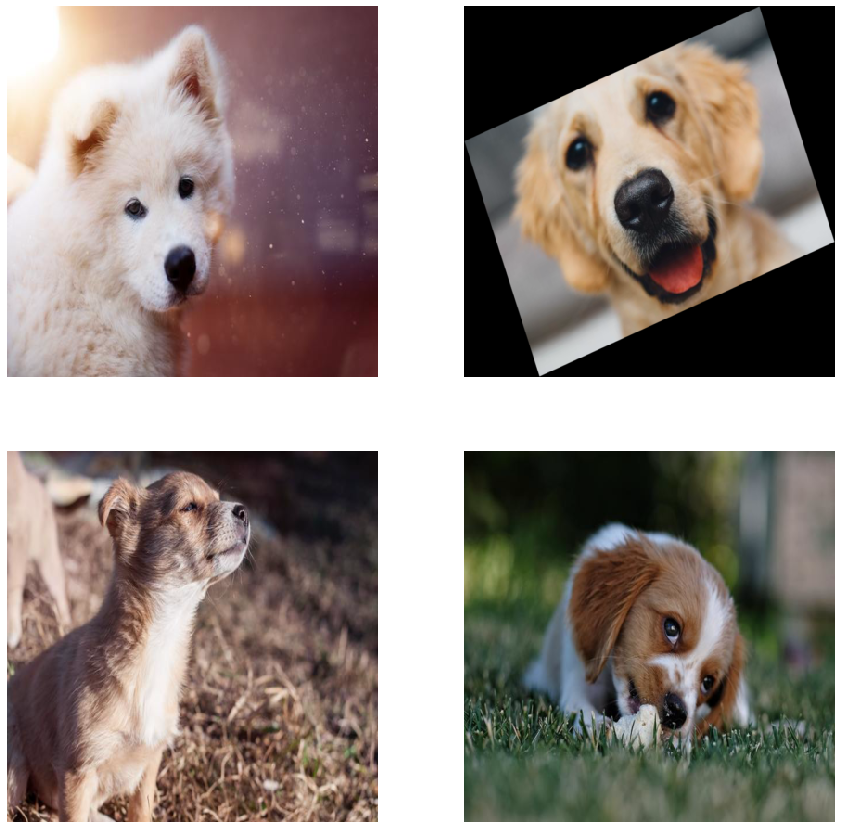
Python 语句和跟踪#
重要的是要记住,只有条件中包含 DataNode 的 if
语句才充当 DALI 操作的条件语句。如果我们用 random.choice([True, False])
语句替换 do_rotate
,则该值将在跟踪期间计算一次,并且 pipeline 图仅知道其中一个分支。当在条件中检测到 DataNode 时,它允许 AutoGraph 转换 if
语句以捕获两个分支并跟踪其中的代码。我们可以通过添加一些打印语句并检查哪些语句会在构建 pipeline 时显示来观察到这一事实。
[12]:
import random
@pipeline_def(
enable_conditionals=True, batch_size=4, num_threads=4, device_id=0, seed=42
)
def pipe_with_dali_conditional():
jpegs, _ = fn.readers.file(device="cpu", file_root="../data/images")
images = fn.decoders.image(jpegs, device="mixed")
dali_random_value = fn.random.coin_flip(dtype=DALIDataType.BOOL)
if dali_random_value:
print("Tracing True branch")
result = images + 10
else:
print("Tracing False branch")
result = images
return result
@pipeline_def(
enable_conditionals=True, batch_size=4, num_threads=4, device_id=0, seed=42
)
def pipe_with_python_conditional():
jpegs, _ = fn.readers.file(device="cpu", file_root="../data/images")
images = fn.decoders.image(jpegs, device="mixed")
dali_random_value = fn.random.coin_flip(dtype=DALIDataType.BOOL)
if random.choice([True, False]):
print("Tracing True branch")
result = images + 10
else:
print("Tracing False branch")
result = images
return result
[13]:
pipe_with_dali_conditional()
Tracing True branch
Tracing False branch
[13]:
<nvidia.dali.pipeline.Pipeline at 0x7f00402e1cf8>
[14]:
pipe_with_python_conditional()
Tracing True branch
[14]:
<nvidia.dali.pipeline.Pipeline at 0x7f0178fed128>
正如我们所见,当条件是 DataNode 时,我们正确地跟踪了两个分支,并且我们的样本将根据相应 sample_idx 的 dali_random_value
的内容进行处理。对于 pipe_with_python_conditional
,我们仅跟踪了其中一个分支,因为该值在跟踪期间获得一次,并且被视为常量。