HSV 示例#
此示例演示了 hsv
算子的用法,该算子操作图像的色调、饱和度和明度(亮度)方面。
简介#
HSV 色彩空间#
HSV 通过分离色调、饱和度和亮度来表示颜色。在此色彩空间中,色调表示为色轮上的一个角度。饱和度从 0(灰度)到 100%(完全饱和的颜色),值从 0(黑色)到 1(全亮度)。有关详细信息,请参阅:Wikipedia。
关于实现的说明#
出于性能原因,DALI 没有使用 HSV 的精确定义,而是通过对 RGB 颜色进行线性(矩阵)运算来近似 HSV 空间中的运算。这大大提高了性能,但代价是适度损失了保真度。
步骤指南#
让我们从导入一些实用工具和 DALI 本身开始。
[1]:
from nvidia.dali import pipeline_def
import nvidia.dali.fn as fn
import nvidia.dali.types as types
batch_size = 10
image_filename = "../data/images"
批大小大于一,以便于在笔记本末尾切换图像。
接下来,让我们实现 pipeline。装饰函数
hsv_pipeline
可用于创建在 CPU 或 GPU 上处理数据的 pipeline,具体取决于device
参数。
[2]:
@pipeline_def()
def hsv_pipeline(device, hue, saturation, value):
files, labels = fn.readers.file(file_root=image_filename)
images = fn.decoders.image(
files, device="cpu" if device == "cpu" else "mixed"
)
converted = fn.hsv(images, hue=hue, saturation=saturation, value=value)
return images, converted
下面的函数用于实际显示 DALI 中 HSV 操作的结果。由于我们设置的 pipeline 返回 2 个输出:修改后的图像和原始图像,因此该函数从输出中获取两者并显示它们。指定了附加标志 (cpu
),以确定 pipeline 输出是来自 CPU 还是 GPU。在后一种情况下,我们必须告诉输出返回数据的 CPU 可访问副本。
[3]:
import matplotlib.pyplot as plt
import matplotlib.gridspec as gridspec
import math
def display(outputs, idx, columns=2, captions=None, cpu=True):
rows = int(math.ceil(len(outputs) / columns))
fig = plt.figure()
fig.set_size_inches(16, 6 * rows)
gs = gridspec.GridSpec(rows, columns)
row = 0
col = 0
for i, out in enumerate(outputs):
plt.subplot(gs[i])
plt.axis("off")
if captions is not None:
plt.title(captions[i])
plt.imshow(out.at(idx) if cpu else out.as_cpu().at(idx))
现在让我们构建 pipeline,运行它们并显示结果。首先是 CPU pipeline
[4]:
pipe_cpu = hsv_pipeline(
device="cpu",
hue=120,
saturation=1,
value=0.4,
batch_size=batch_size,
num_threads=1,
device_id=0,
)
pipe_cpu.build()
cpu_output = pipe_cpu.run()
[5]:
display(
cpu_output, 3, captions=["Original", "Hue=120, Saturation=1, Value=0.4"]
)
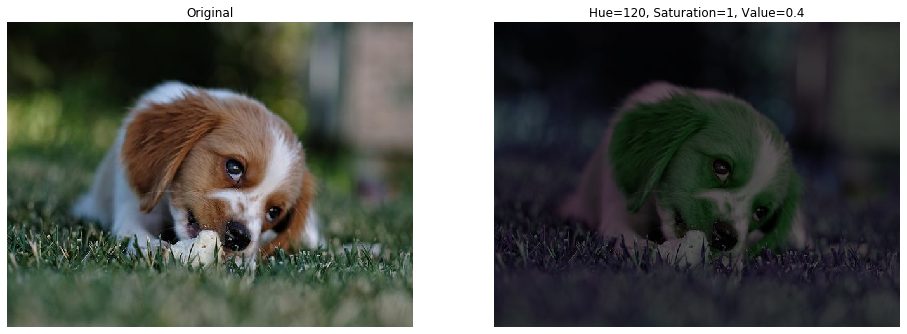
以及 GPU
[6]:
pipe_gpu = hsv_pipeline(
device="gpu",
hue=120,
saturation=2,
value=1,
batch_size=batch_size,
num_threads=1,
device_id=0,
)
pipe_gpu.build()
gpu_output = pipe_gpu.run()
[7]:
display(
gpu_output,
0,
cpu=False,
captions=["Original", "Hue=120, Saturation=2, Value=1"],
)
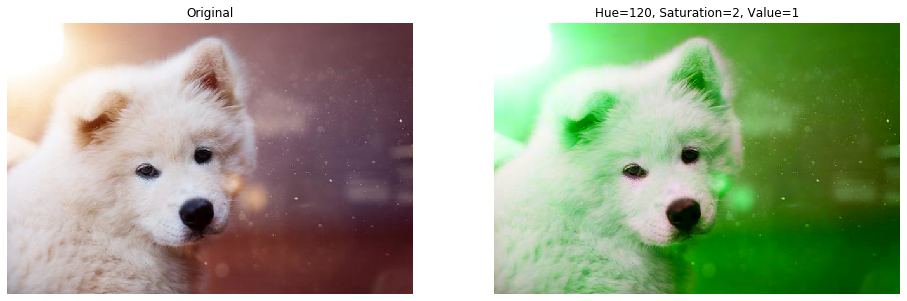
使用 HSV 实现 RandomGrayscale 操作#
作为 Hsv 算子的一个更有趣的示例,我们希望实现类似于 Pytorch 的 RandomGrayscale 变换 - 对于 RGB 输入,将其转换为仍然包含 3 个通道(但值相等)的灰度图像,或者保持不变。
为了实现灰度转换,我们将降低输入的饱和度(将这些样本的 saturation
设置为 0)。如果我们将 saturation
设置为 1,则图像将保持其颜色。
我们可以使用 coin_flip
算子,该算子以可配置的概率返回 0 和 1。我们将使用 coin_flip
生成的值来驱动 hsv
算子的 saturation
参数。
coin_flip
为处理批次中的每个样本生成一个数字,我们可以将其传递给 hsv
中 saturation
参数。由于 coin_flip
返回整数,而 hsv
期望浮点数作为其参数,因此我们还需要使用 cast
转换这些值。
[8]:
def random_grayscale(images, probability):
saturate = fn.random.coin_flip(probability=1 - probability)
saturate = fn.cast(saturate, dtype=types.FLOAT)
return fn.hsv(images, saturation=saturate)
@pipeline_def(seed=422)
def random_grayscale_pipeline():
files, labels = fn.readers.file(file_root=image_filename)
images = fn.decoders.image(files, device="mixed")
converted = random_grayscale(images, 0.6)
return images, converted
现在让我们构建并运行 pipeline。
[9]:
pipe = random_grayscale_pipeline(
batch_size=batch_size, num_threads=1, device_id=0
)
pipe.build()
output = pipe.run()
[10]:
display(output, 0, cpu=False, captions=["Original", "RandomGrayscale"])
display(output, 1, cpu=False, captions=["Original", "RandomGrayscale"])
display(output, 2, cpu=False, captions=["Original", "RandomGrayscale"])
display(output, 3, cpu=False, captions=["Original", "RandomGrayscale"])
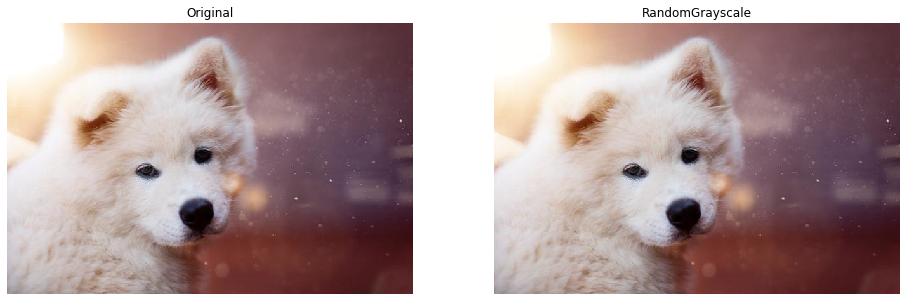
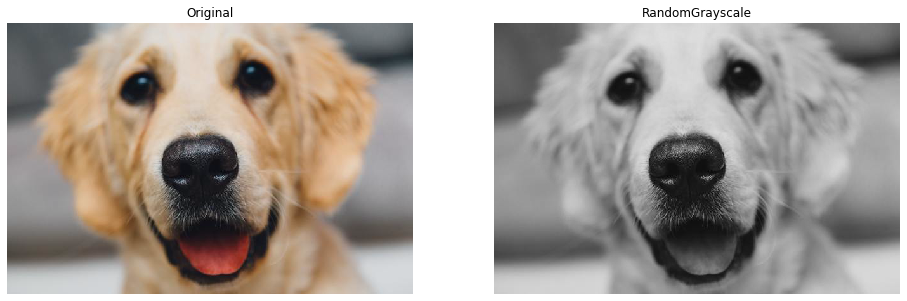
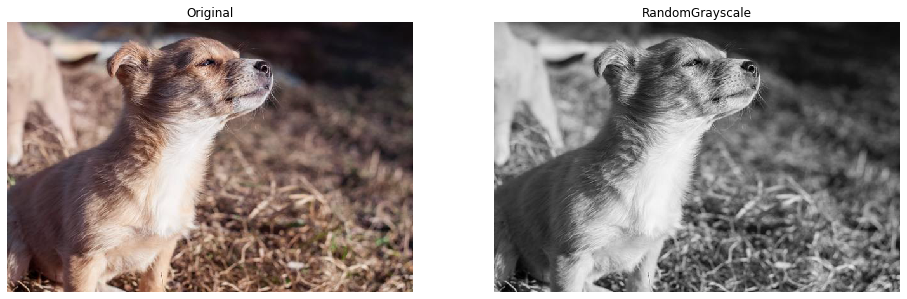
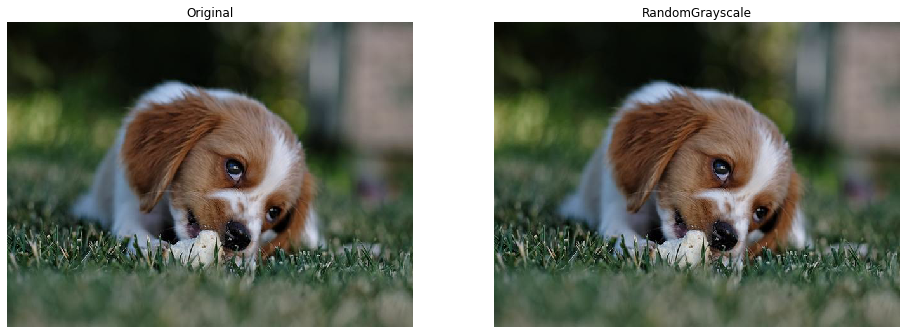