使用 Python 算子处理 GPU 数据#
此示例演示了如何在 GPU 上使用 PythonFunction
算子。有关 Python 算子系列的介绍和一般信息,请参阅 Python 算子 部分。
尽管 Python 算子并非旨在快速运行,但在 GPU 上运行它们可能很有用,例如,当我们想向现有的 GPU pipeline 引入自定义操作时。为此,PythonFunction
系列中的所有算子都有其 GPU 变体。
对于 TorchPythonFunction
和 DLTensorPythonFunction
算子,它们操作的数据格式与 CPU 上的数据格式保持不变,前者是 PyTorch 张量,后者是 DLPack 张量。对于 GPU PythonFunction
,实现函数的输入和输出是 CuPy 数组。
CuPy 操作#
由于 CuPy 数组 API 与 NumPy 中的 API 类似,我们可以实现与 CPU 示例中定义的几乎相同的操作,而无需进行任何代码更改。
[1]:
from nvidia.dali.pipeline import Pipeline
import nvidia.dali.fn as fn
import nvidia.dali.types as types
import numpy
import cupy
def edit_images(image1, image2):
assert image1.shape == image2.shape
h, w, c = image1.shape
y, x = cupy.ogrid[0:h, 0:w]
mask = (x - w / 2) ** 2 + (y - h / 2) ** 2 > h * w / 9
result1 = cupy.copy(image1)
result1[mask] = image2[mask]
result2 = cupy.copy(image2)
result2[mask] = image1[mask]
return result1, result2
使用 CuPy 定义 GPU 函数的另一种方法是编写 CUDA 内核。在这里,我们展示一个简单的内核,用于交错两个图像的通道。有关更多信息,请参阅 CuPy 文档。
[2]:
mix_channels_kernel = cupy.ElementwiseKernel(
"uint8 x, uint8 y", "uint8 z", "z = (i % 3) ? x : y", "mix_channels"
)
警告
当 pipeline 启用条件执行时,必须采取其他步骤来防止 function
被 AutoGraph 重写。有两种方法可以实现此目的
在全局范围内定义函数(即在
pipeline_def
范围之外)。如果函数是另一个“工厂”函数的结果,则工厂函数必须在 pipeline 定义函数之外定义,并使用
<nvidia.dali.pipeline.do_not_convert>
进行装饰。
更多详细信息可以在 nvidia.dali.pipeline.do_not_convert
文档中找到。
定义 Pipeline#
我们定义一个类似于 Python 算子 部分中使用的 pipeline。要将执行从 CPU 移动到 GPU,我们只需要更改算子的设备参数。这也是 PythonFunction
算子的唯一用法差异。
[3]:
image_dir = "../data/images"
batch_size = 4
python_function_pipe = Pipeline(
batch_size=batch_size,
num_threads=4,
device_id=0,
exec_async=False,
exec_pipelined=False,
seed=99,
)
with python_function_pipe:
input1, _ = fn.readers.file(file_root=image_dir, random_shuffle=True)
input2, _ = fn.readers.file(file_root=image_dir, random_shuffle=True)
im1, im2 = fn.decoders.image(
[input1, input2], device="mixed", output_type=types.RGB
)
res1, res2 = fn.resize([im1, im2], device="gpu", resize_x=300, resize_y=300)
out1, out2 = fn.python_function(
res1, res2, device="gpu", function=edit_images, num_outputs=2
)
out3 = fn.python_function(
res1, res2, device="gpu", function=mix_channels_kernel
)
python_function_pipe.set_outputs(out1, out2, out3)
运行 Pipeline 并可视化结果#
我们可以运行 pipeline 并以类似于 CPU 示例的方式显示结果。
注意: 在尝试绘制它们之前,请记住将输出批次移动到主机内存。
[4]:
import matplotlib.pyplot as plt
import matplotlib.gridspec as gridspec
from matplotlib import cm
%matplotlib inline
batch_size = 4
def show_images(image_batch):
columns = 4
rows = (batch_size + 1) // columns
fig = plt.figure(figsize=(32, (32 // columns) * rows))
gs = gridspec.GridSpec(rows, columns)
for j in range(rows * columns):
plt.subplot(gs[j])
plt.axis("off")
plt.imshow(image_batch.at(j))
python_function_pipe.build()
ims1, ims2, ims3 = python_function_pipe.run()
show_images(ims1.as_cpu())
show_images(ims2.as_cpu())
show_images(ims3.as_cpu())
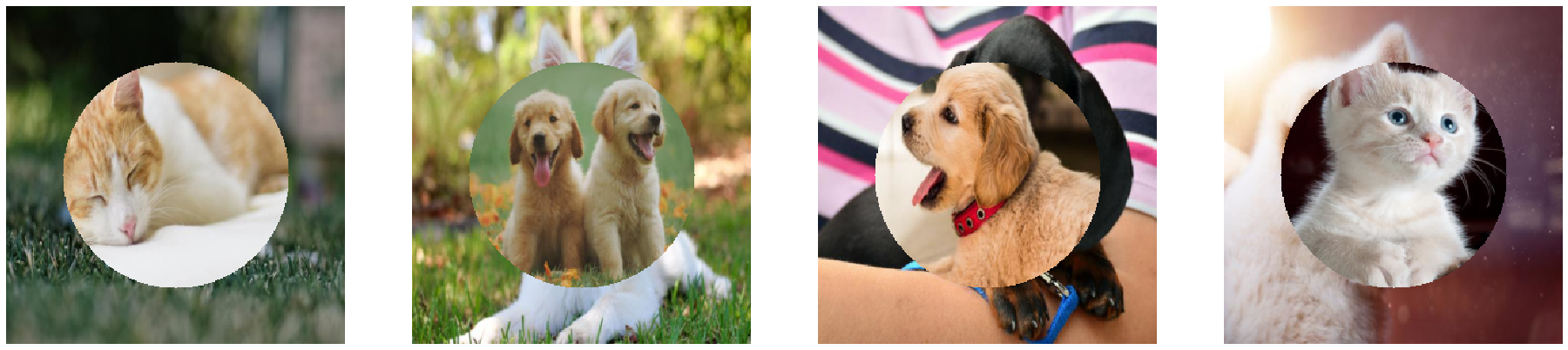
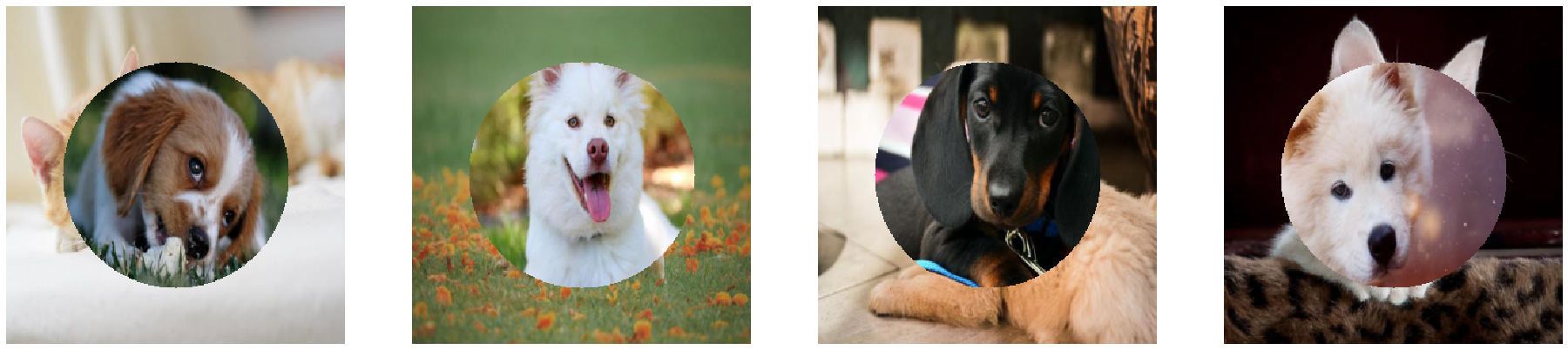
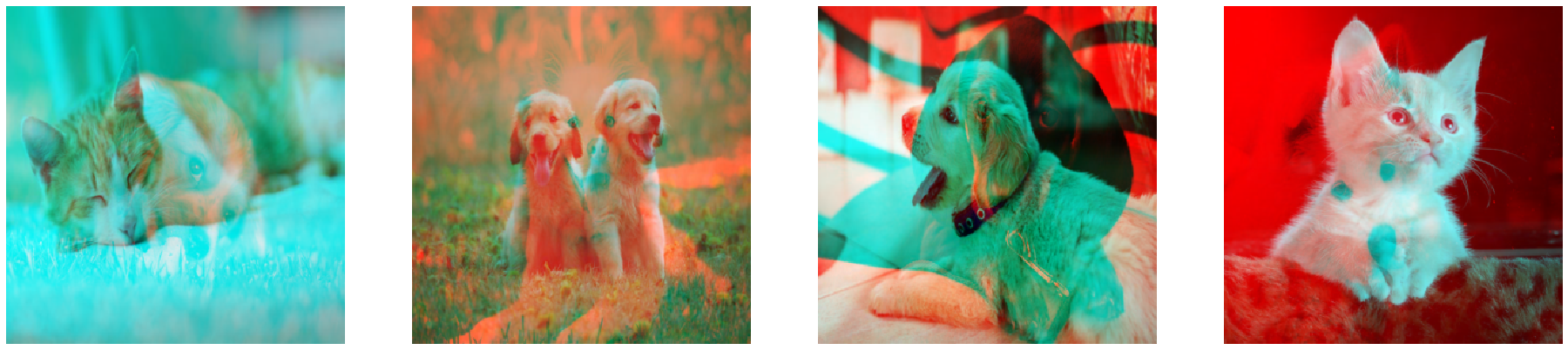
高级:DLTensorPythonFunction 中的设备同步#
当使用 PythonFunction
或 TorchPythonFunction
时,我们不必将 GPU 代码与 DALI pipeline 的其余部分同步,因为同步由算子处理。DLTensorPythonFunction
算子另一方面将设备同步留给用户。
注意: 不同框架和库的同步过程可能有所不同。
例如,我们将围绕先前实现的 mix_channels_kernel
编写一个包装器,该包装器将 DLPack 张量转换为 CuPy 数组并处理流同步。
[5]:
def mix_channels_wrapper(tensor1, tensor2):
array1 = cupy.fromDlpack(tensor1)
array2 = cupy.fromDlpack(tensor2)
result = mix_channels_kernel(array1, array2)
cupy.cuda.get_current_stream().synchronize()
return result.toDlpack()
dltensor_function_pipe = Pipeline(
batch_size=batch_size,
num_threads=4,
device_id=0,
exec_async=False,
exec_pipelined=False,
seed=99,
)
with dltensor_function_pipe:
input1, _ = fn.readers.file(file_root=image_dir, random_shuffle=True)
input2, _ = fn.readers.file(file_root=image_dir, random_shuffle=True)
im1, im2 = fn.decoders.image(
[input1, input2], device="mixed", output_type=types.RGB
)
res1, res2 = fn.resize([im1, im2], device="gpu", resize_x=300, resize_y=300)
out = fn.dl_tensor_python_function(
res1,
res2,
device="gpu",
function=mix_channels_wrapper,
synchronize_stream=True,
batch_processing=False,
)
dltensor_function_pipe.set_outputs(out)
dltensor_function_pipe.build()
(ims,) = dltensor_function_pipe.run()
show_images(ims.as_cpu())
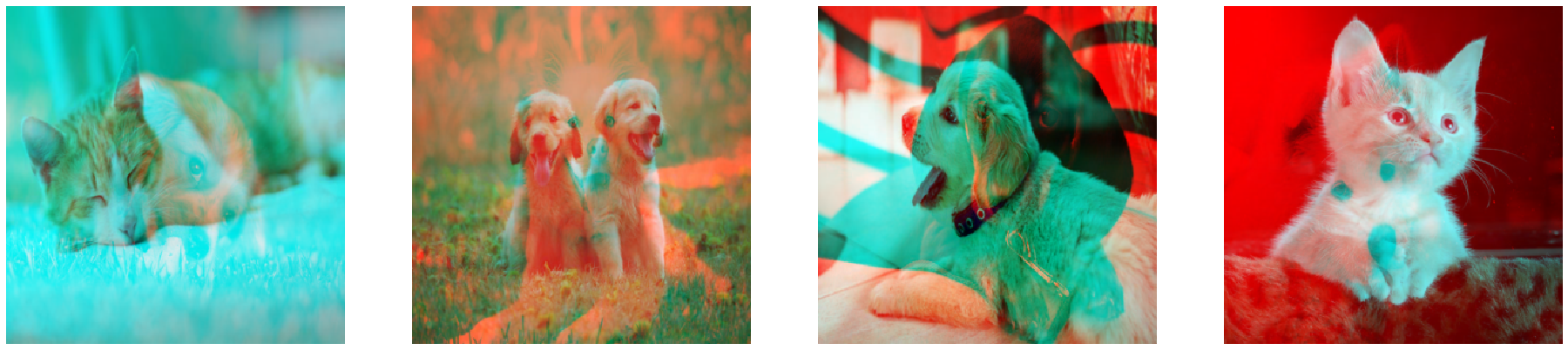
结果与使用 PythonFunction
运行 mix_channels_kernel
后的结果相同。要在 DLTensorPythonFunction
中正确同步设备代码,请确保满足以下条件
在提供的函数开始之前,所有先前的 DALI GPU 工作都已完成。
我们在提供的函数中调度的所有工作在我们返回结果之前都已完成。
第一个条件由 synchronize_stream=True
标志(默认设置为 True
)保证。用户负责提供第二部分。在上面的示例中,通过添加 cupy.cuda.get_current_stream().synchronize()
行来实现同步。