API 使用¶
注意
CUDALibrarySamples
仓库位于 Github 上,其中包含多个示例,展示了如何在各种情况下使用 cuFFTMp。建议读者在阅读本文档时探索这些示例。
与 单进程、多 GPU API 类似,cuFFTMp 中的输入和输出数组分布在多个 GPU 上。这是通过描述符完成的,描述符同时保存指向本地数据的指针和一个枚举,描述数据如何在多个 GPU 之间分布。请注意,在 cuFFTMp 中,每个描述符仅保存指向其 GPU 本地数据的指针,而不指向其他 GPU 的数据。
cuFFTMp 支持两种数据分布。
内置 slabs
这是通过使用 CUFFT_XT_FORMAT_INPLACE
和 CUFFT_XT_FORMAT_INPLACE_SHUFFLED
完成的。这是默认的、优化的数据分布,与单进程 API 相同。考虑一个大小为 XxYxZ
的数组,分布在 n
个 GPU 上。
对于
CUFFT_XT_FORMAT_INPLACE
,前X % n
个 GPU 各自拥有(X/n+1)*Y*Z
个元素,其余 GPU 各自拥有(X/n)*Y*Z
个元素。对于
CUFFT_XT_FORMAT_INPLACE_SHUFFLED
,前Y % n
个 GPU 各自拥有X*(Y/n+1)*Z
个元素,其余 GPU 各自拥有X*(Y/n)*Z
个元素。
请注意,此数据分布不允许元素之间存在步长,并假定使用原地数据布局进行原地变换。这意味着长度为 Z
的实数维度需要填充到 2(Z/2+1)
个元素。
灵活的 slabs 和 pencils
使用 CUFFT_XT_FORMAT_DISTRIBUTED_INPUT
和 CUFFT_XT_FORMAT_DISTRIBUTED_OUTPUT
。这允许用户使用任何 Slab 或 Pencil 数据分布(元素之间可以有任意步长)。虽然高效,但此分布不如前一种分布优化。
结合内置 slab 数据分解使用¶
当使用默认和优化的数据分布时,cuFFTMp 的 API 使用方式与单进程、多 GPU cuFFT API 使用方式类似。以下概述了所需 API 调用的顺序。
// Initialize MPI, create some distribute data
MPI_Init(...)
MPI_Comm comm = MPI_COMM_WORLD;
// Initialize a cuFFT handle
cufftHandle plan = 0;
cufftCreate(&plan);
// Attach an MPI communicator
cufftMpAttachComm(plan, CUFFT_COMM_MPI, &comm);
// Create a plan
size_t workspace;
cufftMakePlan3d(plan, nx, ny, nz, CUFFT_C2C, &workspace);
// Allocate a multi-GPU descriptor
cudaLibXtDesc *desc;
cufftXtMalloc(plan, &desc, CUFFT_XT_FORMAT_INPLACE);
// Copy data from the CPU to the GPU.
// The CPU data is distributed according to CUFFT_XT_FORMAT_INPLACE
cufftXtMemcpy(plan, desc, cpu_data, CUFFT_COPY_HOST_TO_DEVICE);
// Compute a forward FFT
cufftXtExecDescriptor(plan, desc, desc, CUFFT_FORWARD);
// Copy memory back to the CPU. Data is now distributed according to CUFFT_XT_FORMAT_INPLACE_SHUFFLED
cufftXtMemcpy(plan, cpu_data, desc, CUFFT_COPY_DEVICE_TO_HOST);
// Free the multi-GPU descriptor and the plan and finalize MPI
cufftXtFree(desc);
cufftDestroy(plan);
MPI_Finalize();
此代码片段执行以下操作
使用
MPI_Init
初始化 MPI,在 CPU 上创建分布式 2D 或 3D 数组(自然顺序或置换顺序)。(可选)在多 GPU 系统上,使用
cudaSetDevice
选择 GPU。创建空 plan
cufftCreate(&plan)
。将 MPI 通信器
comm
附加到 plan,指示 cuFFT 启用多进程功能。cufftMpAttachComm(plan, CUFFT_COMM_MPI, comm)
(可选)附加流
cufftSetStream(plan, stream)
。制作 plan。这将创建一个用于大小为
nx*ny*nz
的 3D 复数到复数变换的 plancufftMakePlan3d(plan, nx, ny, nz, CUFFT_C2C, workspace)
这将创建一个用于大小为
nx*ny
的 2D 复数到复数变换的 plancufftMakePlan2d(plan, nx, ny, CUFFT_C2C, workspace)
由于 cuFFTMp 仅支持无步长的原地变换,因此不应使用
cufftMakePlanMany
。请注意,这是一个集体调用,应由 MPI 通信器中的所有进程调用。分配 GPU 内存以使用
cufftXtMalloc(plan, desc, CUFFT_XT_FORMAT_INPLACE)
保存数据。CUFFT_XT_FORMAT_INPLACE
指示数据根据自然顺序分布。CUFFT_XT_FORMAT_INPLACE_SHUFFLED
可用于分配置换顺序的数据。内存分配在desc->descriptor->data[0]
中。使用
cufftXtMemcpy(plan, desc, cpu_data, CUFFT_COPY_HOST_TO_DEVICE)
将数据从 CPU 复制到 GPU。请注意,数据也可以从一开始就在 GPU 上初始化,而使用cufftXtMemcpy
仅仅是一个方便的函数。可以通过使用设备内存指针desc->descriptor->data[0]
直接访问 GPU 数据。
运行变换
cufftXtExecDescriptor(plan, desc, desc, CUFFT_FORWARD)
此时,数据以置换顺序分布。如果需要,可以执行逐元素操作、滤波、反向变换等。
使用
cufftXtMemcpy(plan, cpu_data, desc, CUFFT_COPY_DEVICE_TO_HOST)
将数据从 GPU 复制回 CPU。这不会更改数据分布。如果 GPU 数据根据CUFFT_XT_FORMAT_INPLACE
(或CUFFT_XT_FORMAT_INPLACE_SHUFFLED
)分布,则 CPU 数据也是如此。释放描述符
cufftXtFree(desc)
。销毁 plan
cufftDestroy(plan)
。使用
MPI_Finalize
最终确定 MPI。
警告
cufftMakePlanMany
和 cufftXtMakePlanMany
不应与 cuFFTMp 一起使用。如果使用它们,任何步长信息都将被忽略。
最后,您可以按如下方式构建和运行
包含
cufftMp.h
头文件编译应用程序并链接到 cuFFTMp (
libcufftMp.so
)、NVSHMEM host (libnvshmem_host.so
) 和 CUDA 驱动程序 (libcuda.so
)。请注意,不允许静态链接到 NVSHMEM (libnvshmem.a
)。另请注意,libcufftMp.so
包含单进程和多进程 cuFFT 功能,因此无需链接到libcufft.so
。假设${CUFFTMP_HOME}
和${NVSHMEM_HOME}
分别是 cuFFTMp 和 NVSHMEM 的安装目录mpicxx app.cpp -I${CUFFTMP_HOME}/include -L${CUFFTMP_HOME}/lib -L${NVSHMEM_HOME}/lib -lcufftMp -lnvshmem_host -lcuda -o app
在运行时,确保 NVSHMEM 引导程序 (
nvshmem_bootstrap_mpi.so
) 存在于LD_LIBRARY_PATH
中export LD_LIBRARY_PATH=${NVSHMEM_HOME}/lib:$LD_LIBRARY_PATH
如有必要,通过将
NVSHMEM_SYMMETRIC_SIZE
环境变量设置为例如 10GB,来增加为 NVSHMEM 保留的内存量export NVSHMEM_SYMMETRIC_SIZE=10G
使用 MPI 运行应用程序,例如:
mpirun -n 2 ./app
结合自定义 slabs 和 pencils 数据分解使用¶
cuFFTMp 还支持以 3D boxes 形式表示的任意数据分布。考虑一个 X*Y*Z
全局数组。3D boxes 用于通过指示子部分的下角和上角来描述此全局数组的子部分。通过将 boxes 与进程关联,可以描述一种数据分布,其中每个进程拥有全局数组的连续矩形子部分。例如,考虑一个 2D 案例,全局数组的大小为 X*Y = 4x4
和三个 boxes,描述为 box = {lower, upper}
Box 0 = { {0,0}, {2,2} }
(绿色)Box 1 = { {2,0}, {4,2} }
(蓝色)Box 2 = { {0,2}, {4,4} }
(紫色)
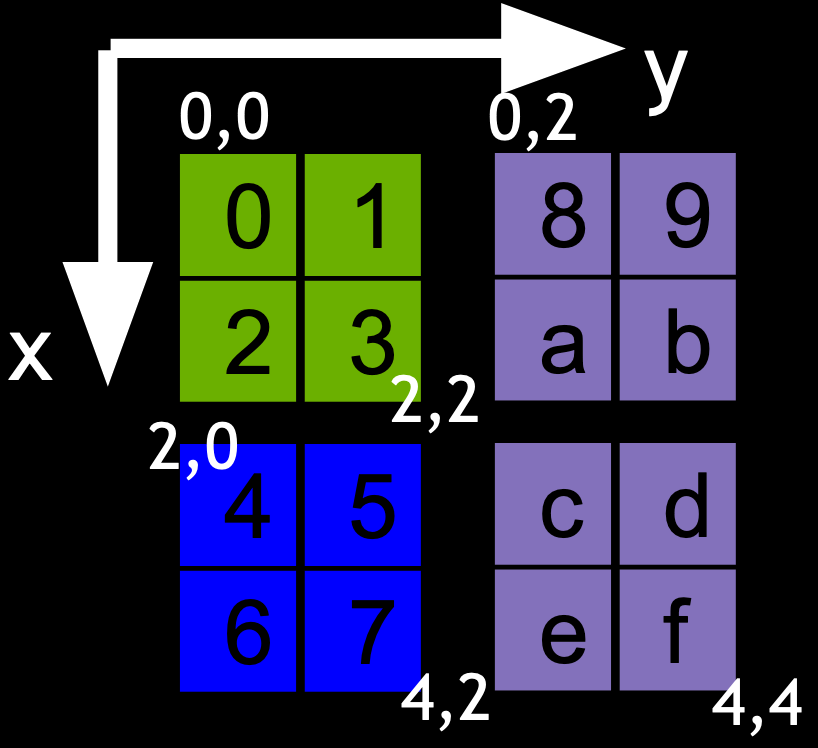
通过将 box 0 与进程 0 关联,box 1 与进程 1 关联,box 2 与进程 2 关联,这创建了全局 4*4
数组在三个进程之间的数据分布。相同的过程可以推广到 3D 数组。
此逻辑可用于推广上一节的用法,并使用任何数据分布。这需要使用 cufftXtSetDistribution(plan, rank, lower_input, upper_input, lower_output, upper_output, strides_input, strides_output)
函数。使用此函数,cufftXtExecDescriptor(plan, ...)
可以接受数据分布类型为 CUFFT_XT_FORMAT_DISTRIBUTED_INPUT
或 CUFFT_XT_FORMAT_DISTRIBUTED_OUTPUT
的描述符。对于给定的 plan
,
CUFFT_XT_FORMAT_DISTRIBUTED_INPUT
表示描述符中的数据根据(lower_input, upper_input)
分布,并且内存中的数据布局由strides_input
描述;CUFFT_XT_FORMAT_DISTRIBUTED_OUTOUT
表示描述符中的数据根据(lower_output, upper_output)
分布,并且内存中的数据布局由strides_output
描述。
请注意,此外,
R2C
plans 仅支持CUFFT_XT_FORMAT_DISTRIBUTED_INPUT
,即(lower_input, upper_input)
应描述实数数据分布,而(lower_output, upper_output)
应描述复数数据分布;C2R
plans 仅支持CUFFT_XT_FORMAT_DISTRIBUTED_OUTPUT
,即(lower_input, upper_input)
应描述实数数据分布,而(lower_output, upper_output)
应描述复数数据分布。
这意味着 R2C
后跟 C2R
plan 的正确用法是
cufftXtSetDistribution(plan_r2c, 3, real_lower, real_upper, complex_lower, complex_upper, real_strides, complex_strides)); cufftXtSetDistribution(plan_c2r, 3, real_lower, real_upper, complex_lower, complex_upper, real_strides, complex_strides)); ... cufftXtExecDescriptor(plan_r2c, desc, desc, CUFFT_FORWARD); ... cufftXtExecDescriptor(plan_r2c, desc, desc, CUFFT_INVERSE);
总的来说,对于 C2C plan,这将导致以下结果
// Initialize MPI, create some distribute data MPI_Init(...) MPI_Comm comm = MPI_COMM_WORLD; // Create plan cufftHandle plan = 0; cufftCreate(&plan); cufftMpAttachComm(plan, CUFFT_COMM_MPI, &mpi_comm); // Initialize input and output data distribution descriptors long long int lower_input[3], upper_input[3], lower_output[3], upper_output[3], strides_input[3], strides_output[3]; ... // initialize above arrays cufftXtSetDistribution(plan, 3, lower_input, upper_input, lower_output, upper_output, strides_input, strides_output); // Make the cuFFT plan size_t scratch; cufftMakePlan3d(plan, nx, ny, nz, CUFFT_C2C, &scratch); // Allocate a multi-GPU descriptor cudaLibXtDesc *desc; cufftXtMalloc(plan, &desc, CUFFT_XT_FORMAT_INPLACE); // Copy data from the CPU to the GPU. // The CPU data is distributed according to CUFFT_XT_FORMAT_DISTRIBUTED_INPUT cufftXtMemcpy(plan, desc, cpu_data, CUFFT_COPY_HOST_TO_DEVICE); // Compute a forward FFT cufftXtExecDescriptor(plan, desc, desc, CUFFT_FORWARD); // Copy memory back to the CPU. Data is now distributed according to CUFFT_XT_FORMAT_DISTRIBUTED_OUTPUT cufftXtMemcpy(plan, cpu_data, desc, CUFFT_COPY_DEVICE_TO_HOST); // Free the multi-GPU descriptor and the plan and finalize MPI cufftXtFree(desc); cufftDestroy(plan); MPI_Finalize();
创建空 plan
cufftCreate(&plan)
将 MPI 通信器附加到 plan,指示 cuFFT 启用多进程功能
cufftMpAttachComm(plan, CUFFT_COMM_MPI, comm)
(可选)附加流
cufftSetStream(plan, stream)
描述数据分布。首先定义输入和输出 boxes,描述此进程拥有的全局数组的子部分。然后,调用
cufftXtSetDistribution
以向 cuFFT 注册相应的数组long long int lower_input[3], upper_input[3], lower_output[3], upper_output[3], strides_input[3], strides_output[3]; ... // initialize above arrays cufftXtSetDistribution(plan, 3, lower_input, upper_input, lower_output, upper_output, strides_input, strides_output);
使用以下命令制作 plan
cufftMakePlan3d(plan, nx, ny, nz, CUFFT_C2C, &scratch)
这将创建一个用于(全局)大小为
nx*ny*nz
的 3D 变换的 plan。分配 GPU 内存以保存数据。
CUFFT_XT_FORMAT_DISTRIBUTED_INPUT
指示数据根据input_box
分布cufftXtMalloc(plan, desc, CUFFT_XT_FORMAT_DISTRIBUTED_INPUT)
使用以下命令将数据从 CPU 复制到 GPU
cufftXtMemcpy(plan, desc, cpu_data, CUFFT_COPY_HOST_TO_DEVICE)
请注意,使用
cufftXtMemcpy
是可选的。相反,数据可以直接在设备上初始化和操作。另请注意,cufftXtMemcpy
复制整个 CPU 缓冲区,实际上忽略了除第一维步长之外的所有步长。运行变换
cufftXtExecDescriptor(plan, desc, desc, CUFFT_FORWARD)
将数据从 GPU 复制回 CPU。此时,数据根据
CUFFT_XT_FORMAT_DISTRIBUTED_OUTPUT
(即output_box
)分布。cufftXtMemcpy(plan, cpu_data, desc, CUFFT_COPY_DEVICE_TO_HOST)
使用
cufftXtFree(desc)
释放描述符使用
cufftDestroy(plan)
销毁 plan
结合 NVSHMEM 使用¶
要将 cuFFTMp 与 NVSHMEM 分配的缓冲区一起使用,用户需要:
初始化和最终确定 NVSHMEM;
使用
nvshmem_malloc
和nvshmem_free
分配和释放内存;在 plan 上调用
cufftXtSetSubformatDefault(plan, subformat_forward, subformat_inverse)
以指示cufftExecC2C
或类似 API 期望的数据分布。subformat_forward
将是正向变换的输入数据分布,而subformat_inverse
将是反向变换的数据分布。
将此代码片段与上一示例中使用描述符的代码片段进行比较,请参阅 结合内置 slab 数据分解使用。直接调用 NVSHMEM API 的代码需要链接到 <0xE2><0x80><0x8B>libnvshmem_host.so<0xE2><0x80><0x8B> 和 libnvshmem_device.a
。
// Initialize MPI, create some distribute data
MPI_Init(...)
MPI_Comm comm = MPI_COMM_WORLD;
// Initialize NVSHMEM
nvshmemx_init_attr_t attr;
attr.mpi_comm = (void*)&comm;
nvshmemx_init_attr(NVSHMEMX_INIT_WITH_MPI_COMM, &attr);
// Initialize a cuFFT handle
cufftHandle plan = 0;
cufftCreate(&plan);
// Attach an MPI communicator
cufftMpAttachComm(plan, CUFFT_COMM_MPI, &comm);
// Create a plan
size_t workspace;
cufftMakePlan3d(plan, nx, ny, nz, CUFFT_C2C, &workspace);
// Allocate NVSHMEM memory
cuComplex* gpu_data = (cuComplex*)nvshmem_malloc(...);
// Indicate default data distribution expected by cufftExecC2C
cufftXtSetSubformatDefault(plan, CUFFT_XT_FORMAT_INPLACE, CUFFT_XT_FORMAT_INPLACE_SHUFFLED);
// Copy data from the CPU to the GPU.
// The CPU data is distributed according to CUFFT_XT_FORMAT_INPLACE
cudaMemcpy(gpu_data, cpu_data, ..., cudaMemcpyDefault);
// Compute a forward FFT
// CUFFT_FORWARD means the input is CUFFT_XT_FORMAT_INPLACE and the output is CUFFT_XT_FORMAT_INPLACE_SHUFFLED
// CUFFT_INVERSE would mean that the input is CUFFT_XT_FORMAT_INPLACE_SHUFFLED and the output is CUFFT_XT_FORMAT_INPLACE
cufftExecC2C(plan, gpu_data, gpu_data, CUFFT_FORWARD);
// Copy memory back to the CPU. Data is now distributed according to CUFFT_XT_FORMAT_INPLACE_SHUFFLED
cudaMemcpy(cpu_data, gpu_data, ..., cudaMemcpyDefault);
// Free the NVSHMEM memory and the plan and finalize NVSHMEM and MPI
nvshmem_free(gpu_data);
cufftDestroy(plan);
nvshmem_finalize();
MPI_Finalize();
Reshape API¶
cuFFT 公开了一个分布式 reshape API,该 API 允许重新分布使用 3D boxes 描述的数据,而无需使用 cuFFT plan、描述符或 cufftXtMemcpy
。其用法遵循通用的 cuFFT 用法,但具有新的句柄类型。
// Initialize MPI MPI_Init(...); MPI_Comm comm = MPI_COMM_WORLD; // Initialize input and output data distribution descriptors long long int lower_input[3], upper_input[3], lower_output[3], upper_output[3], strides_input[3], strides_output[3]; ... // initialize above arrays // Allocate reshape, attach MPI communicator cufftReshapeHandle handle; cufftMpCreateReshape(&handle); cufftMpAttachReshapeComm(handle, CUFFT_COMM_MPI, &comm); // Build reshape cufftMpMakeReshape(handle, sizeof(int), 3, lower_input, upper_input, lower_output, upper_output, strides_input, strides_output); // Create a stream cudaStream_t stream; cudaStreamCreate(&stream); // Retreive workspace size size_t workspace; cufftMpGetReshapeSize(handle, &workspace); // Allocate NVSHMEM memory void *src = nvshmem_malloc(input_size * sizeof(int)); void *dst = nvshmem_malloc(output_size * sizeof(int)); void *scratch = nvshmem_malloc(workspace); // Apply reshape CUFFT_CHECK(cufftMpExecReshapeAsync(handle, dst, src, scratch, stream)); // Cleanup nvshmem_free(src); nvshmem_free(dst); nvshmem_free(scratch); cufftMpDestroyReshape(handle); cudaStreamDestroy(stream); MPI_Finalize();
创建空 reshape 句柄,
cufftMpCreateReshape(handle)
附加 MPI 通信器,
cufftMpAttachReshapeComm(handle, CUFFT_COMM_MPI, comm)
制作 reshape
cufftMpMakeReshape(handle, sizeof(int), 3, lower_input, upper_input, lower_output, upper_output, strides_input, strides_output);
(lower_input, upper_input)
(每个进程上一个)描述输入分布,而(lower_output, upper_output)
描述输出分布。strides_input
和strides_output
(每个进程上一个)描述内存中的数据布局。element_size
是单个元素的大小(以字节为单位)。这是一个集体调用。使用
cufftMpGetReshapeSize(handle, workspace)
检索工作区大小(以字节为单位)。使用 NVSHMEM 分配工作区
使用以下命令执行 reshape
cufftMpExecReshapeAsync(handle, dst, src, workspace, stream)
这是一个流有序的集体调用。
dst、src、workspace
都应是指向对称堆、NVSHMEM 分配的内存缓冲区的指针。请注意,这与 MPI 不同,在 MPI 中,dst、src、workspace
将是指向 cudaMalloc 分配内存的常规指针。另请参阅 NVSHMEM 关于对称堆的文档 此处。此外,当一个 GPU 开始在流中执行cufftMpExecReshapeAsync
时,目标内存缓冲区dst
和暂存缓冲区workspace
应在所有其他 GPU 上准备就绪,以防止出现竞争条件。这可以通过例如在 API 之前放置同步点(如nvshmemx_sync_all_on_stream(stream)
)来实现。使用
cufftMpDestroyReshape(handle)
清理并销毁 reshape。
注意
这些函数需要使用 NVSHMEM 来分配内存。 nvshmem_malloc
和 nvshmem_free
可用于此目的。有关更多信息,请参阅 NVSHMEM 文档 和 cuFFTMp 中的 NVSHMEM 内存缓冲区。
请注意,对称堆 NVSHMEM 分配的缓冲区具有与本地缓冲区不同的语义。特别是,dst、src、workspace
都应指向在所有 GPU 上分配的同一对称对象。这与传递指针到基于消息传递的库(如 MPI)时不同,在 MPI 中,指针仅指向本地对象。
辅助函数¶
与单进程、多 GPU API 类似,cuFFTMp 提供了辅助函数来帮助管理多个 GPU 内存。它们的用法与单进程情况类似。
cufftXtMalloc
用于在多个进程中的多个 GPU 上分配内存。它接受一个参数,指示数据分解。cufftXtMemcpy
用于在 CPU 和 GPU 之间或 GPU 之间复制内存。其用法取决于数据传输的源和目标cufftXtMemcpy(plan, dst, src, CUFFT_COPY_HOST_TO_DEVICE)
将分布式 CPU 数据复制到 GPU,保持分布不变。这意味着 CPU 数据必须以与 GPU 数据相同的方式分布。cufftXtMemcpy(plan, dst, src, CUFFT_COPY_DEVICE_TO_HOST)
将分布式 GPU 数据复制到 CPU,保持分布不变。这意味着 CPU 数据将以与 GPU 数据相同的方式分布。cufftXtMemcpy(plan, dst, src, CUFFT_COPY_DEVICE_TO_DEVICE)
可用于将 GPU 数据从CUFFT_XT_FORMAT_INPUT_SHUFFLED
重新分布到CUFFT_XT_FORMAT_INPUT
(或反之亦然)。这对于将置换数据转换回自然顺序以便于后处理非常有用。
cufftXtFree
用于释放描述符